Podcast
Special Episode: Health Care in CA, Panel 1 – AI in Health Care
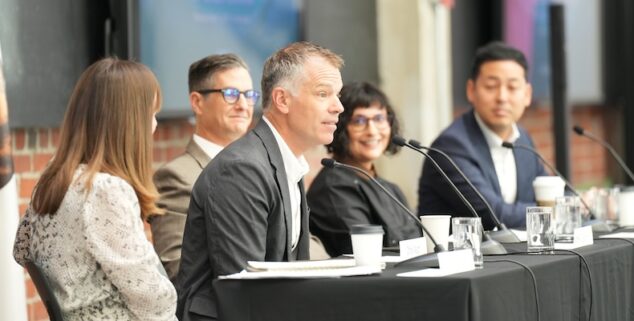
CAPITOL WEEKLY PODCAST: This Special Episode of the Capitol Weekly Podcast was recorded live at Capitol Weekly’s conference HEALTH CARE IN CALIFORNIA, which was held in Sacramento on Thursday, October 3, 2024
This is PANEL 1 – AI IN HEALTH CARE
Panelists: Kara Carter, California Health Care Foundation; Sam Chung, California Life Sciences; Matthew D. Solomon, MD, Kaiser Permanente; Chris Waugh, Sutter Health
Moderated by Samantha Young, KFF Health News
This transcript has been edited for clarity.
SAMANTHA YOUNG: Thank you. Hi, everybody. My name is Samantha Young. I’m a deputy California editor with KFF Health News. And welcome to our first panel, AI in Health Care. I’m your moderator, as Rich said. And I’m just going to get right to introducing our guests because we have a lot of good things to talk about here. I’m thinking it’s going to be a very robust conversation on a technology that just has the potential to revolutionize health care. But it also carries some very big risks when it comes to equity, privacy, just to name a few.
So starting to my very left, we have Sam Chung. He is vice president of state government relations at California Life Sciences. Kara Carter is senior vice president of strategy and programs at the California Health Care Foundation. We have Matthew Solomon, who is a cardiologist at Kaiser Permanente. And we have Chris Waugh from Sutter Health. He is the chief innovation officer.
So welcome all, and thank you very much. I’m going to start with our first question, which I would love all of you to answer. And I just wanted to set the stage. When we talk about AI and kind of give people an idea of what is it? How is it already being used, kind of like demystify it all for for everybody who’s in the room. And for those of you who are watching on zoom. Maybe we’ll start with Sam.
SAM CHUNG: Sure I can. Great question. There’s so many different areas where we can highlight where AI is currently used for the life sciences. I think one of the most prevalent ways is in our drug discovery process. Fundamentally, we are trying to make new medicines that don’t exist. So we’re going from molecules to new medicines, which is very, very difficult to do.
What AI has been able to do for our scientists and researchers is to make that drug discovery process a lot more efficient and more effective, and it makes our drug discovery much more expansive. In the human body, there are 37 trillion cells, thousands of different types of cells in the human body. 20,000 genes within the human genome, and millions of variant combinations. And so when you’re looking at all these different molecules and how they may react to certain compounds, there is a ton of trial and error. There’s a reason why, when we’re trying to develop a new drug, on average, it takes about ten years, a decade. $2.6 billion per medicine with a 92% failure rate. Now AI is able to reduce all those numbers.
“Ai is able to, just by recording your voice, be able to tell us whether you’re susceptible to diabetes and be able to prescribe treatment just based off of analyzing your voice…. This has been a huge game changer” – Sam Chung
And so it allows scientists and researchers to really get rid of all the repetitive and mundane things you are able to rely on, rely on AI technology to really speed up that discovery process, so that scientists can focus on important parts of the R&D process, rather than on repetitive exercises. Because of the number of combinations that you’re constantly checking through the trial and error process. And so it is a true game changer when it comes to our companies being able to bring treatments to patients faster.
A different another area where we see AI presently being used in a very positive way is in clinical trials by our companies. Using AI, we’re able to look at different geographies. We’re able to look at electronic medical records that are de-identified, and we’re able to really optimize the profile of the individual that we’re going after and where we’re going to do the site selection to get the right combination of people representing different demographics, so that we’re getting the best clinical trial data possible, so that whatever medicines do come out are effective for all people, regardless of skin pigmentation and genetics.
And so those those are two, I think, big ways where AI is really revolutionizing the life sciences space. And then just to give a couple examples and there’s there’s so many… But when it comes to the world of radiology, when by the time the human eye can can detect pancreatic cancer, it’s a death sentence. There’s there’s no way by then it’s too late. But with AI, you’re able to detect pancreatic cancer better than the best radiologists in the world. So it gives you enough time to be able to treat that pancreatic cancer. And so that that is one way in the imaging space where we’re really seeing AI changing the game for patients around the world.
And then just just another example. You know, when somebody when we’re screening for diabetes, it’s usually an invasive process because you’re needing to take blood out of the person and then running a test. Ai is able to, just by recording your voice, be able to tell us whether you’re susceptible to diabetes and be able to prescribe treatment just based off of analyzing your voice. And in places like India. This has been a huge game changer, being able to diagnose and be able to provide treatments for those who are susceptible or beginning to feel the effects of diabetes. And so those are just two, two examples that I want to highlight. There’s so many and we’ll get into them as we have this panel discussion.
SY: All right. Great. Thank you.
KARA CARTER: I’m hard to follow that. I’m excited by all of those new treatments. As somebody who’s had cancer myself, I’m excited to hear what we’re doing. In the spirit of demystifying, I would just say that where Sam started with some of the excitements and innovations that are happening today, I would note that AI has been with us for a long time. I mean, we’re on probably 70 years of AI, and I hear it talked about in kind of 2 or 3 waves where you started in the 1960s with you know, computers and machines that were programmed to do very narrow tasks or very specific things. And then we had a wave in the late 90s, early 2000s where you know, computers were where machine learning was really beginning. And we really began to see its impact on health care there. And what we talk about really, in the last three years or so is this third wave of generative AI, which takes what we have done historically to a different. It just takes it to a different level. It’s less around being programmed to do a narrow task, and more around machines that are learning themselves and continually improving themselves.
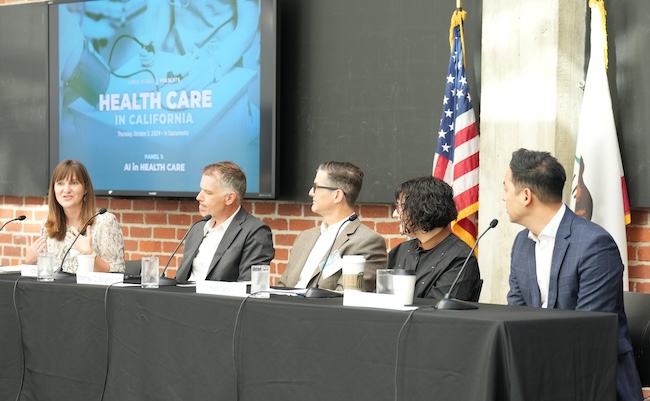
Pane 1: AI in Health Care. L-R: Samantha Young, KFF Health News; Chris Waugh, Sutter Health; Matthew D. Solomon, MD, Kaiser Permanente; Kara Carter, California Health Care Foundation; Sam Chung, California Life Sciences. Photo by Joha Harrison, Capitol Weekly.
So when we walk around the state and talk to healthcare leaders about what they’re doing with AI, both machine learning and predictive AI, and, you know, the exciting features of generative AI that we’re all talking about, we hear from plans, providers and also community clinics and CBOs, the kinds of things that they’re implementing today.
This isn’t a horizon for the future. This is this is what is happening right now that is leading to better health outcomes and improved health care in our system.
So for the plans who have been at this for a very long time, probably since second wave that I mentioned, they’ve been deep in using AI on a lot of back office use cases. So claims adjudication, operational efficiencies, reducing readmissions, patient segmentation, marketing, reaching out to patients, a whole list of administrative cases that they’ve been using. They talk about. Providers…. I’m sure we’ll hear from my colleagues many clinical use cases that are extremely exciting. I hear about them in diagnosing sepsis. I hear early warnings in ICUs. I hear a ton of radiology and pathology use cases, and I hear diabetic retinopathy use cases, all kinds of screening.
But then I also hear things that just help make the patient experience better or the provider experience better. And those would be things like translation services, things like patient communications. After the after office visit summary that you get you know, coming to you in language that you understand rather than language that your provider would use is a really great one that we hear about a lot. And then a ton of just administrative lift. And we hear that from our community clinics, too. So you know, ambient scribing and coding are probably the number one use cases. Those are hugely attractive because they solve provider needs that are felt today. They’re, you know, they’re not adding workload. They’re they’re helping ease providers burden, allowing people to spend more direct time in patient care.
And then we hear a few other just really interesting use cases from a, you know, health services that are perhaps not always thought of as being at the forefront, excuse me, of innovation. So we hear from CBOs how community health workers are using generative AI applications today to meet the needs of their communities where they’re at sometimes actually because they don’t come with the existing complexity of systems to build on. They’re able to start start fresh and start scratch and build things that are really novel.
MATTHEW SOLOMON: That was wonderful. I agree with everything that has been said before.
So it’s wonderful to be here. Thank you for inviting me. I help lead our what we call our Augmented Clinical Intelligence Program within Kaiser Permanente Northern California. And I think I if you think about what AI is from a very simple definition, it’s really using a computer to do a complex task.
And as was noted, this has been done for decades. And in healthcare it’s been done for decades. And it’s it’s really embedded in healthcare’s DNA. In fact, in our organization in our founding back in the 40s and 50s, Doctor Sidney Garfield, who was one of our Kaiser Permanente founders, wrote in Scientific American how the health care system can get overwhelmed with patients. And we need to use a computer to help figure out to to sort patients from the very sick to the worried well or the well, and, and how do we identify people that we can do preventive care on and who needs the most attention. So allocating resources to risk. People have been thinking about that since the 50s.
And you know, I think of AI as really a spectrum. We use a ton of risk calculators or clinical calculators in healthcare. There’s.. I have an app on my phone that has hundreds of them. And those are really one form of AI. They’re a simple algorithm. I mean, somebody developed a model somewhere based on statistical technique that maybe is not as advanced as machine learning, but still was fairly advanced. And we use that to help guide us in how to treat patients.
So I’ll give you one example that was developed about 20 years ago in our system. And that was a neonatal sepsis calculator. So to help identify newborns who might be at risk for getting sepsis very early on and providing preventive antibiotics, and there was some concern that we were across, you know, the health care giving way too many antibiotics to these newborn babies. And so some talented pediatricians and scientists in our system developed a model to help identify which patients are most likely to develop early onset sepsis. And they are the ones that should get preventive antibiotics. And that’s now used all over the world and has reduced the rate of antibiotic use, as well as blood cultures, which are very difficult in newborn babies by about 50%.
Now that model just has, you know, about 10 to 20 inputs. Fast forward 10 to 15 years. One of our flagship AI models that we’re really proud of is we call the Advanced Alert Monitor, or it’s another name for it is a Deterioration Index. So it’s been known for decades that patients, some patients in the hospital are likely to deteriorate and either have… either die unexpectedly or need an or emergency transfer to the ICU. And so using our 21 hospitals and billions of data points, we created a very sophisticated algorithm to kind of in real time suck in all that data – The vital signs, the labs, the nursing notes – to identify which patients are likely to have a bad outcome in the next 12 hours.
“All healthcare organizations are excited about this because physician burnout has just been a massive, massive problem.” – Matthew Solomon
But that’s not really where I think the hard work in AI is done. The model building tends to be easy. It’s getting it into practice and the workflow to actually use it well. That is the hard part. You know, it’s been said that if the building, the model is one unit of work, it’s about 19 units of work to get it into the workflow appropriately. And that’s one of been been one of our really big learnings.
And so that model is now deployed across our 21 medical centers. It’s going on right now. But we had, you know, many learnings from this where you, you don’t want to automatically use AI. There’s a lot of talk like “our computer is going to make the decisions.” No. The computer sends an alert to a team of virtual nursing team that looks in the chart and decides, is this a patient in whom we need to activate a rapid response and send a nurse or a doctor to the bedside? Because if you if you flag a lot of patients who are, like, falsely, you know, who don’t need the care, the doctors and the teams on the ground aren’t going to buy into it.
So and there’s dozens and dozens of those examples. And our team shepherds these models from either a research idea all the way through to development, you know, with a very standardized process to make sure we’re doing it responsibly. But, you know, it was never called IA five years ago.
And I think what changed the game is this third phase that Kara mentioned, and that’s the introduction of generative AI and ChatGPT that, you know, put AI. And the fact that, you know, my mother knows what ML means, right? And it’s, but it’s really just a spectrum. There are more sophisticated analytic techniques that can take in more information and perform a cognitive task. And I think in healthcare, you know, this is a mission-driven field. Our goal is to improve health. It’s not to grab eyeballs or make dollars. And so you know, we’re really excited about these tools to improve health. They’re, you know, they’re absolutely necessary for any population health that’s been ongoing for decades to eliminate health care disparities. And I think, as you noted, to really improve the patient and provider experience.
So I see patients for 50% of my time. And the ambient dictation is probably the first generative AI tool that we have rolled out. And we were so excited about this. And I know all other all healthcare organizations are excited about this because physician burnout has just been a massive, massive problem. And the electronic medical record, which is incredible, has really cut into the doctor patient relationship. And, you know, I try not to stare at the screen while I’m asking patients questions and typing it in, but ambient dictation is truly incredible. It’s synthesizing the conversation.
We’ve rolled it out in a more rapidly than I’ve ever seen technology rolled out in our organization, and we’ve done it in a very thoughtful, transparent way. Patients, you know, are, you know, there’s full disclosure to patients about whether they want to use it and how the data are used. I had a patient, you know, say they didn’t want it to use it just a couple of days ago, so we didn’t use it. But it’s transformative to be able to restore that doctor patient relationship. And I’m really looking forward to the future with where there are many, many more examples where I think that that’s where providers and patients are going to be helped in overall health will improve.
CHRIS WAUGH: Samantha. I wondered if we’d get through this first question….
SY: The questions in the first question. So yeah.
CW: Couple of quick comments. One is I, I agree with everything said so far. I was trained at Ido, which is a design firm, to think about first order principles and look from the outside in. So I’m actually going to start with the patient and not talk about a health system for a second. There’s over 350,000 health and wellness apps in the App Store.
Most raise your hand in the room if you’re tracking something on your health, if it’s your sleep or your steps or anything. Okay, so you’re doing that. Historically, if you tried to bring that to your doctor, they wanted nothing to do with it for good reason. Most of the reasons Matt just described, overwhelm, burnout. I don’t know what to do with this information. How is it clinically relevant, etc..
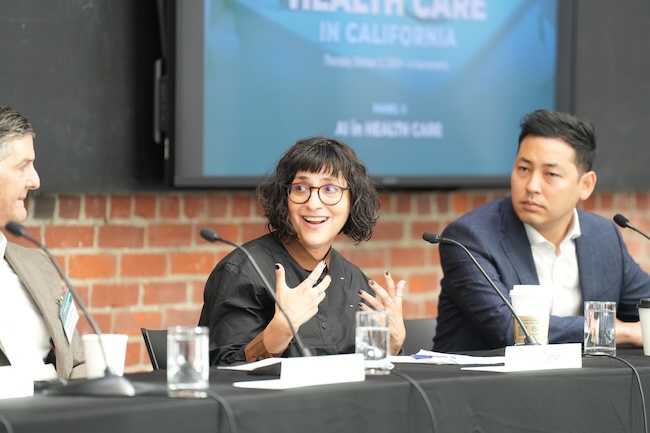
Pane 1: AI in Health Care. L-R; Matthew D. Solomon, MD, Kaiser Permanente; Kara Carter, California Health Care Foundation; Sam Chung, California Life Sciences. Photo by Joha Harrison, Capitol Weekly.
Four major things happened. One is the electronic medical record came to life. That’s an almost killed doctors because it’s so difficult to put all the information in. The beautiful outcome of that is we’ve now had years and years of information gathering. Now you have the internet, all the apps we just described, all the wearables you’re wearing, producing on a second by second basis, millions of bits of new information about how people are living, moving, exposing themselves to different things. And for health, that is a tremendous opportunity, as described by Sam, where we’re putting together really unusual combinations of things to figure out this is where diseases come from; These are the patterns that we can pick up on that could help in those issues, even down to our people, socially isolated. Are they not socially isolated? Things of that nature.
The other thing that came to life that is not commonly described outside the medical field is PubMed. Pubmed is where all research lives in public domain.
I’ll give you an example now of the main issues in health care. You can’t access health care. So the majority of people can’t get into health care. So what’s happened now with AI is we’ve put an incredibly powerful tool in their hands, even if they could never access health care. Those that do access health care. There are misdiagnoses. There are people that go undiagnosed, and there are people, as Sam described, that come in that could be better matched. Clinical trials, for example, AI matching patients to the right clinical trial happening somewhere in the world at any given time is one of the most beautiful arrangements that we are going to be able to see. And it’s starting to take shape right now. That’s beautiful.
“I’m not telling you that ChatGPT is going to successfully diagnose you. I’m just saying that it certainly has the potential to get awfully close.” – Chris Waugh
We believe that our doctor has that all in their head. That’s what we’d love to think is they’re aware of every single clinical trial that goes on anywhere in the world. And we also believe that they stay up all night obsessing about what you told them, looking and reading on every last minute bit of literature that came out up to last night, so that you’re getting the best possible treatment.
The truth is, now we can. That is what I is allowing them to do, is to sit almost side by side with the best person in their class that has the best knowledge about anything, and get counsel and advice and feedback that can be brought back to you in a bespoke way. Maps perhaps all the way down to your genetic sequence and your particular cell. So I think that’s amazing.
Equity. We also talk about equity. I think there’s a big opportunity to actually use AI to point out to us where we’re not being equitable. That’s a massive use case. So yes, AI has inherent bias. And yes, AI itself can tell us where that bias exists to identify and express to us, “you’re missing something,” or a patient is expressing something that is not part of the research trial, but should be. And then we’re back to the loop with Sam again around why aren’t we running more trials on different races, different styles and different locations, etc.?
This morning I’m going to just make it really real. Oh, here’s a misdiagnosed example that I heard recently. A woman’s child had trouble talking, wasn’t walking well, and had occasional headaches. She went and saw specialist after specialist. This was not in our system. This is a NOM [National Outcomes Measures] story and took all the medical records, that and the notes that she was given, which you can now get. Put it into ChatGPT with a little bit of that PubMed research that she could find and spread out the diagnosis of Tethered Cord Syndrome. And it was right.
So this is really powerful stuff. Now, I’m not saying and for the record, alongside Matt and others, I’m not telling you that ChatGPT is going to successfully diagnose you. I’m just saying that it certainly has the potential to get awfully close. And there are many other tools. The issue we have with ChatGPT is it takes so much information, much of which we don’t know the source of. So the power of large language models built in a clinical context is we can track back the source of, say, the 55 million de-identified records that were used in drug discovery. We can figure. We know where that came from. And so that’s really helpful in the tracing back option. Right now this morning Matt mentioned ambient listening is beautiful. You walk into your doctor’s appointment, start talking. And Matt, if Matt was my doctor and I’d love it if you were Matt.
MS: Hopefully you don’t need me.
CW: We do need you and we’re going to get to that too. Is Matt’s actually sitting there looking at me, listening to me. In fact, it worked so well. Matt can say, “is there anything else?” which is normally what a doctor is cringing to ask a patient, is there anything else? Because there’s definitely something else. And they know the clock is ticking right behind them and their day is stacked just as yours are, but back to back to back to back. And the charting is happening at night. And they’re sitting there at dinner with their families, trying to remember everything that happened that day, that they need to go back into the chart and document tonight. Right now, at the end of that encounter, in that in that conversation with Matt, we could have even switched from Mandarin to English, back to English, or back to Mandarin and got the clinical accuracy with the note automatically populated. And Matt is just approving the note. That’s it. That changes the physician’s life and it changes the patient’s life. And we look at what we call Cognitive Burden. That means the amount of emotional load and cognitive load we’re putting on physicians. And yes, we’re saving some time. But the more important measure is we’re reducing the cognitive load.
The second we haven’t heard about yet is imaging. Imaging is where the action is. This morning in our system, we’ve scanned over 600,000 images for lung nodules. The way that AI works is the radiologist reads the screen. The AI also reads the screen and will if it sees something that was gone undetected, will simply put it back in line. We know we’re catching cancers that we weren’t catching before.
So that’s now. Next is breast. Breast cancer. Breast cancer erly detection. There’s a 1% error rate in radiology, which is incredible. All of us walked around this morning and made errors. There’s a 1% error rate, which is mind boggling. But you don’t want that to be you. And AI would be there as the backup, the support. We hear a lot that this is not artificial intelligence. It’s augmented intelligence, meaning it’s working alongside the practitioners to support them.
Also, today is tomorrow, actually we go into a tool called Synthesia. So tomorrow will be writing scripts in a tool called Synthesia, which is allowing us to educate people on what is hypertension. Within a matter of an afternoon we could record that in multiple languages, and people will be able to play it back at. Tell it to me like I’m a first grader. Tell it to me like I’m a fifth grader. And they won’t do that with their doctor. Because at the end of the day, Matt’s really smart. And if I’m with Matt and I don’t know what a lung nodule is, I don’t want to waste his time. And I’m kind of embarrassed. But as soon as I leave the session, I can get everything I want down to a level of understanding that I can grasp and know what the next move is. So these are really powerful use cases, and I think we’re just scratching the surface right now.
SY: Absolutely. And thank you all. This has been such a great overview. And you touched on so many different topics. And so I’m going to kind of ask if we can rapid fire answer the next few questions. And one of the things that Chris, you mentioned is that I can have a bias. And I’m curious how what are some of the, the concerns or fears about AI? We talked you talked about a lot of the positives it can bring.
What are some of the worries and what kind of guardrails should people be thinking about? And if we could just kind of maybe try, I know it’s going to be hard to try to keep the answer to maybe two minutes, so we can try to get through a few of the questions and just I don’t know who wants to take that first. You don’t all have to answer the question.
MS I’m happy to start. So bias and equity are hugely important in healthcare overall, and they’ve come to the fore in AI, but it’s not really new, actually, when it comes to thinking about how we use data or clinical algorithms to treat patients.
I mentioned risk calculators previously, so I’m a cardiologist. One of the things we see a lot of patients with a condition called atrial fibrillation, it can cause blood clots that can cause strokes. So we use a simple risk calculator that has about six elements your age or your sex, whether you have hypertension, diabetes, a couple other things to determine if you need a strong blood thinner or not. This was based on a small sample in in a European country, but it’s used all over the world. Is that is the background stroke rate in where it was developed the same across all the regions of America? No. Was there any way to sort of customize this model for different areas, to make it more attuned to the local population, so that there wasn’t over/under treatment? No.
Now, because AI is synthesized, or the current analytic techniques that we call AI are analyzing such large swaths of data, the concern about where was this model trained and what data was it trained on has very appropriately come to the table. And I think institutions like ours and Chris’s and others are have in place very robust systems to make sure that any model that we put into practice has gone through a very rigorous checklist, including a bias and equity assessment, which makes sure does the outcome that we’re trying to predict. Does the model work well in men and women in white and black patients of different age groups? And we make sure that every model that we work on before it’s developed or if someone brings to our group, goes through that assessment. But to be honest, that really wasn’t done 10 or 20 years ago because the capacity to do it wasn’t there.
So that’s one way in which I think the data in which a model is built on can affect how that model is used translates to, well, there are things you can do to make sure it’s working on your local population.
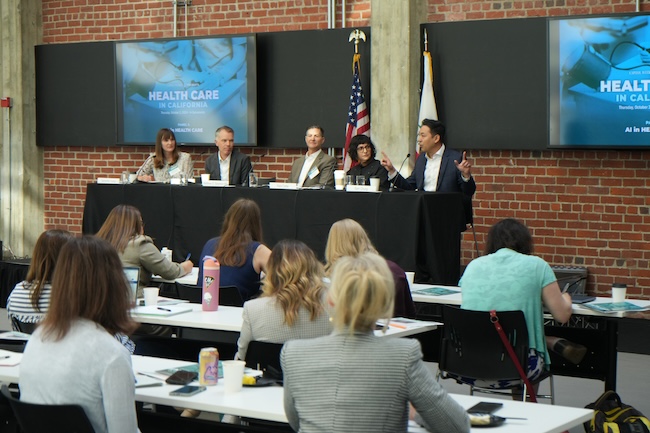
Pane 1: AI in Health Care. L-R: Samantha Young, KFF Health News; Chris Waugh, Sutter Health; Matthew D. Solomon, MD, Kaiser Permanente; Kara Carter, California Health Care Foundation; Sam Chung, California Life Sciences. Photo by Joha Harrison, Capitol Weekly.
But I also want to say that I, you know, I think, as Chris noted, has a lot of opportunity to reduce disparities in health care. So, for example, in colon cancer, historically, black patients had a much higher rate of colon cancer and were much more likely to die of colon cancer. And using computer techniques, which we can call AI to do outreach, population health, preventive screening, and to understand the patterns and ways in which we can encourage people to get screened, changing the ways that we can get that they can get screened. We reported a couple of years ago in New England Journal of Medicine that disparities for colon cancer in our system had been eliminated. White and black patients had the same outcomes. They were much improved.
AI tools can also work in under-resourced settings where there are a lot of disparities. So there’s a big one of there’s a big grant that we are administering for the Gordon and Betty Moore Foundation called Aim High.And one of our recipients works in San Ysidro, a federally qualified health agency. And they’re using an AI tool that does diabetic retinopathy screening. So then the patients come in to see their doctor. They don’t have ophthalmologists there to do it. Not enough. And so they can come in, do the screening, get the results right away.
So a lot of opportunities to both make sure the models are working well for everybody and improving health for everybody.
SC: I would love to jump in and talk about guardrails. There are a ton of guardrails currently in place via the federal government. Just in the last year since the White House issued its executive order on AI. There’s been 25 different guidances, rules and reports issued by 13 different federal agencies, including the FDA and Health and Human Services. And so we the life sciences. And I’m going to speak specific to the life sciences. We we have a sacred relationship with the FDA. The FDA has been regulating AI technology in health care products for over 25 years, starting with MRIs, CAT scans. Those are AI embedded technologies that the FDA is vetting and approving, and they’re specifically looking at bias issues and whether the underlying technology has been corrected and and calibrated to make sure that that has been mitigated.
And so it’s when we’re talking about guardrails, we always want to balance it with not inhibiting innovation. And the FDA is in the best position to do that because of the iterative process we have with the FDA. Whenever they issue reports and guidances, Our companies have an opportunity to comment and provide feedback on those regulations, and the FDA will adjust accordingly. Not only that, but they do audit our companies. And so they come in and make sure that our AI technology and the and the data that we’re entrusted with, that the proper guardrails on that data.
And so there is a long standing relationship. The FDA has approved over 700 medical devices that have AI as an essential component of that device. And so I think having an agency that’s constantly thinking about… that’s apolitical, that’s non-political subject matter experts… who are working hand in hand with our companies to make sure we’re taking the right risk based approaches to AI is huge.
And so when we talk to our companies, we really want to have that risk based approach. There are AI technologies that really are aren’t doing having consequential impacts on patients. So if it’s employees wanting to use AI technology to communicate with each other or share data, very, very low risk, as we get closer to the patient, the risks are elevated and that’s where our companies will slow down their process in adapting certain AI technologies.
And so one of the most telling examples of this is a while back we noticed that Frito-Lay, you know, the potato chip company, they were using AI imaging technology to manufacture, you know, beautiful looking potato chips. I mean, and they, they, they were ahead of life sciences companies, not because we don’t have the technical capabilities to do it, we obviously do. But because of the potential risk to patients, we will slow that process down to make sure we have the proper guardrails in place.
And so it really is an ecosystem where we are working with regulators, subject matter experts, looking just not only just at the bias in technologies, but making sure we have international compliance with Europe, with Canada and others who also have AI regulatory frameworks. And so I think us being able to work with the federal government on that is important. And this is why we do have concern when states start jumping in and saying, hey, we’re going to regulate AI as well, because there’s we’re not we’re concerned that when you have a 50 state patchwork of AI regulations, that makes it very difficult to advance innovation to the benefit of patients.
KC: I’d love to jump in on this one, too.
SY: I have one quick one last question before we go to our audience questions. So I do want you guys to jump in, but if you could keep it short, that would be great.
KC: I just wanted to take a step back because I heard your question is around what the barriers are or risks are, of which bias is a very well-known one. And for me, it’s helpful to take a quick step back and say, I think we’re talking about two, two broad kinds of things.
One is about the technology itself, like, does it work? Does it have a one? What is our risk tolerance for the technology working? Is it 1%? Is it 5%? Is it zero? Is it higher than for a human physician? Is it lower? So does it work? That’s one kind of bucket. The other bucket, which I actually think is almost as, if not more important, is how do we implement it and how do we use it?
And so that’s where the questions, some of the questions on bias come in. I quite agree with Matthew by the way. We have written in bias to our clinical guidelines for 100 years in medicine. It’s not new, we are cementing them or we have a concern about cementing them in our algorithms that reflect our historic inequities. But the historic inequities are not new. They’ve always been there.
And the questions for me on implementation also come to governance. How do we how are we governing this within our not just in our state or federal levels, but within our institutions? And how are we pricing it in a way that makes sure that not just, you know, institutions like those at this table can can deploy this technology and use it.
But, you know, you mentioned San Ysidro. So our community, our federally qualified health centers, our community clinics, our primary care use cases, how do we make sure that those folks where we go for our care every day, have access to implementing this technology?
“Medi-cal is the insurer of first resort for more than a third of California. So we’re not talking about a small number or something off on the side.” – Kara Carter
And finally, you know, all improvements in medicine and otherwise move at the speed of trust with patients and with communities. You know, we put out a survey last year. It tells us, you know, I mean, my glass of water here is a little bit more empty than full. And that’s broadly where the public is right now. So, you know, roughly 50% of Californians welcome AI in healthcare and in their in health care providers and 50% don’t.
And to capture all of the improvements that we’re talking about at this table, we have to that needle has to move. And how do you do that. You do that through involvement. You do it through consenting processes. You do it through clear and open communication with patients and community. And I think that’s critical to to getting past some of the barriers and concerns that you that you raised.
CW: Yeah, just really quickly, I think that health care as we know it right now has inherent bias already in it. So if we look at the train models, they are responding to data that they’re trained on and fed into. So if we go full circle back to Sam’s opening remarks, if we can radically accelerate the discovery process and the research process and even the FDA approval process, we will fast… We’ll have our we’ll have a fighting chance at taking the bias out and making a more equitable health system. So I don’t think AI is the is the villain in this story. It is the our ability to move as fast as possible to take the biases out.
SY: Thank you. This question is for for Kara. And it kind of comes from this this question on the inequities. And I’m curious what your take is on AI’s potential to enhance and improve the state’s health care safety net, especially for communities served by Medi-Cal, which we know is a more than a third of Californians. Right. It’s big. It’s really big. So how do we ensure that poor communities aren’t left behind, especially when we know that big technologies, AI, they’re expensive. And you know, with EHR, we know that those were some of the communities that last kind of got them right or. So how do we how do we make sure that this is equitable as.
KC: Yeah. I so appreciate you asking that question. I mean, first I’ll just start where you ended, which is Medi-Cal is big. It’s not you know, we talk about a safety net. To me, a safety net makes it sound like it’s the last resort. Medi-cal is the insurer of first resort for more than a third of California. So we’re not talking about a small number or something off on the side.
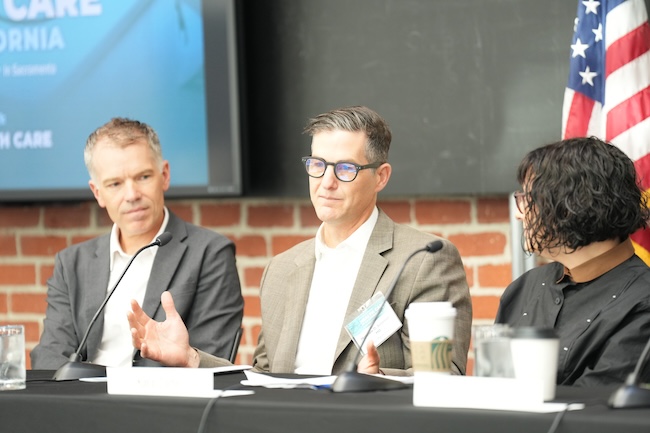
Pane 1: AI in Health Care. L-R: Chris Waugh, Sutter Health; Matthew D. Solomon, MD, Kaiser Permanente; Kara Carter, California Health Care Foundation. Photo by Joha Harrison, Capitol Weekly.
And actually, you know, Californians that carry a Medi-Cal card or plan card, that is a Medi-Cal card. Use the same institutions that that are represented on this panel. You know, the opportunity for Medi-Cal to me, is the same as the opportunity for all Californians. Right?
We’re talking about, hopefully, you know, in my glass, half full world, a world where AI helps our physicians and our other providers free up time, spend more time facing patients, confirm or augment their ability to make better decisions, and to get there. There are there are, I hear consistently from leaders of our safety net clinics and public hospitals that they have needs that are not yet being met to allow them to implement and move forward with this technology.
What is that? It’s everything from risk mitigation. I mean, we talked a little bit about regulation. You know, a a small communication or a small letter can, you know, can raise all kinds of concerns with, you know, who is who is at risk, right. So if it goes wrong, is the company is the vendor that I’m using at risk? Is my institution at risk? Who is at risk? So clarity on that is really important.
I hear a lot about pricing and pricing that is currently targeted towards commercial institutions, and not targeted at a level that yet makes sense. Hopefully more competition, more innovation brings pricing down, and I hear a lot about the opportunity for peer learning and peer to peer convenings and best practices. So on implementation, what have you done? What can I learn? What can I do? You see a lot of that in the safety net rather than competitive behavior and just a big need for it right now.
And I guess the last two things I would say is consistent with many other things that we hear in where we are in this point of time, making sure that our reimbursement and payment models reflect, reflect the opportunity that AI brings. So that we have we have refreshed ways of paying providers that make sense in an AI world.
And then I would end with where I started in the last question, which is, is how we build patient trust and community trust, and how we build space for involvement and conversation.
SY: Great. Well thank you. I think now we’re going to open it up to questions, and we have some mics that will be floating around the room. So if you would like to ask a question raise your hand. Or and we also will have questions coming in on zoom. Anybody in the room have a question? And if you could let us know please who your question is directed to that would be helpful.
AUDIENCE QUESTION: Joan Allen with SEIU, United Healthcare Workers, And to the to the panel broadly with with as particularly generative AI, we’re bringing new companies into this space who may be startups may not have as much of that institutional feel for how sensitive we need to be with patients. And saw the example two weeks ago of the Texas attorney general settling with an AI company over a deceptive practices case where that AI company had misrepresented the error rate in their product to Texas hospitals that then deployed their technology in hospitals.
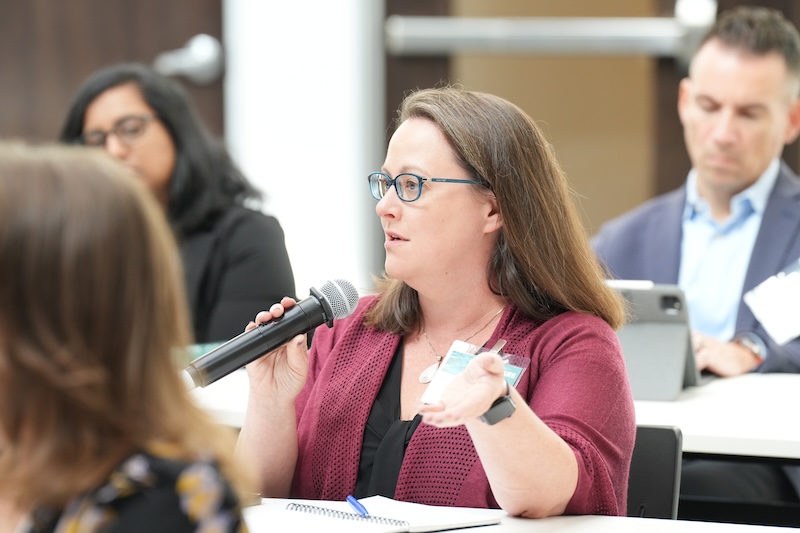
Panel 1: AI in Health Care. Audience question. Photo by Joha Harrison, Capitol Weekly.
How are you thinking about the interaction between your systems and those AI companies that may or may not have a track record, that may or may not have transparent practices, and where, particularly with with a generative AI model, it is very difficult to see under the hood of the model.
CW: So that’s a great question. And I think you’re right that it’s an exciting time in the kind of vended commercial space for AI products, and it can be a little bit of the Wild West, too, because the ability and capacity to build tools is getting easier. And so separating kind of the wheat from the chaff is going to be really important.
“I ride my bike to work and I ride right through the heart of San Francisco. The time I feel the safest is next to a self-driving car. But the self-driving car has to be better than the best driver in the world in order to prove itself that it’s viable for broader distribution.” – Chris Waugh
So one of the things I think, to answer your question, when you think about the model life cycle there’s a very… we have and I think all big health systems have this, and this is getting to be kind of the standard of care and responsible AI. When you think about the model life cycle or all the steps in the checklist that you do before you put anything in place, and the part of that life cycle where what you described should have been caught is probably what we call silent validation, where if you’re interested in a solution and you want to bring it into your system, you don’t just unleash it on patients. Nothing touches a patient or provider or gets into what we call operations until you’ve really evaluated it rigorously, internally and made sure that the performance is is meeting, you’re seeing that in a silent mode where it’s, you know, you’re running it in the background to try and understand how it’s working, but you’re not actually using the results. You’re kind of evaluating it internally. And I could imagine in our system where that’s where that might have been caught. So I think having those best practices for responsible AI, ensuring that that’s the case making that kind of the community standard is one of the important ways to help prevent cases like you described.
RICH EHISEN: Well, I have one for you. And please correct me if I have the context wrong here, because I may have, but you’re mentioning the impact of AI to be able to take a look at a community and then kind of generate responses based on what they know about that particular community. How does changing demographics work into all of that? I mean, is it something that evolves in real time? Because, as we know, sometimes community demographics can change very rapidly.
[Sorry, what’s the question specifically?]
RE: Well, the question is how does how confident are you that if you’re going to take in community demographics as a part of an AI’s ability, or using an AI system to diagnose or have a general idea of what a particular condition may how it may be related to where that person lives. But, you know, with demographics that can change rapidly. How rapidly can AI adapt to those kinds of changes? Sorry, I should have asked that more clearly.
KC: I think I think you’re asking a question about training data and how you continually train. Is that. Do I have it? Yes.
RE: That’s. Yes. Thank you. You’ve sussed it out better than I did.
KC: Thank you. I mean, I think that’s an excellent question, right? And that, to me, is inherently tied to the points that I was making about community trust. So when you when you if you start from the assumption which I do that consenting with patients and communities on what data you’re using is important, how you build up those partnerships with communities to make sure that you are building trust in the way that you take in the way that you use and deploy data is, for me, the first step to getting to a place where you’re able to continually bring in the right data from communities.
And we actually have really good examples of this across the state, actually, right in your backyard here at UC Davis, they’ve they’ve built an entire program in their population health management systems that is this pretty unique partnership between a population health, the Diversity, Equity and Inclusion office and the office for IT to make sure that they are building the trust they need with communities to be able to bring in the right data to address the evolving needs of communities and patient populations in a in a way that you know, that kind of innovation does move at the speed of trust, and the speed of trust is sometimes slow.
So you’re trying to what I’ve watched them navigate very carefully is trying to balance the need to slow down, to build that trust with the need to speed up so that you are continually innovating in the way that Chris describes.
CW: Yeah. I think just to add to that is the the tmagnitude of the training will not be specific to a single location. Right. So I’ve seen some models. Sam’s probably seen it. And you guys have all seen things like these where the model trained was 55 million de-identified medical records, teasing out, you know, any demographic shift you could dream of.. and using that, then knowing, hey, we are picking up a pattern on a demographic shift of who’s in our area. And now we have this really deep pool of insights globally from patients like that with a history that we could only – we could never imagine. Right. And and having all that at our fingertips.
So I think that comes down to how do we pick up on the pattern that the demographic is shifting. And that’s pretty solid. That’s pretty simple. I think that’s a blend of, you know, census data plus who’s coming in and just, you know, there’s easy tracking of who are we seeing and what does the environment look like. But then how we can respond to that I think is powerful.
I also want to address the prior question is… I ride my bike to work and I ride right through the heart of San Francisco. The time I feel the safest is next to a self-driving car. But the self-driving car has to be better than the best driver in the world in order to prove itself that it’s viable for broader distribution. If we had if we had all cars driving at what the best self-driving cars are doing now, we would see almost zero fatalities. But the bar for those self-driving cars is exponentially higher than the driving test you and I took to get our driver’s license.
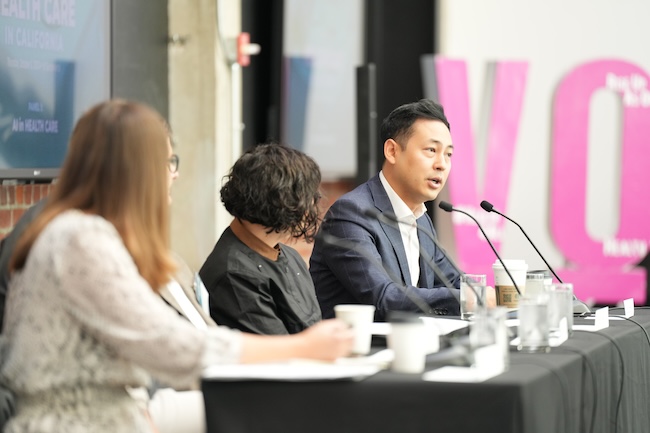
Pane 1: AI in Health Care. L-R: Samantha Young, KFF Health News; Chris Waugh, Sutter Health; Matthew D. Solomon, MD, Kaiser Permanente; Kara Carter, California Health Care Foundation; Sam Chung, California Life Sciences. Photo by Joha Harrison, Capitol Weekly.
And this is also true with the startup community. While systems can’t do it by themselves, the talent doesn’t exist in a single place. This is why we put an innovation center in the middle of San Francisco, because we knew those startups were going to be around us. We know all the checks and balances that Matt described are there. Is it zero risk? It’s never zero risk. Are there errors? Yes, there are errors. Should it always be better than a human if you just took the accumulated data? How much a human makes an error versus the AI? It should be running circles around the human error.
So a real case example for you right now is… and does it solve a bigger problem? We’re working with a stealth startup right now in cognitive behavioral therapy AI. It’s trained cognitive behavioral therapy. We know as a term the variation in cognitive behavioral therapy is as good as the random person you get assigned to. It’s not very consistent, but we know the term. We’ve heard the term right. So the model is trained to the best in class cognitive behavioral therapy models that exist in the world. Raising the bar for anyone that delivers that kind of therapy.
But the beauty to the person is instead of maybe you never can get access to a therapist, which is true for most people… but even if you did to have a companion, which is, I had this interesting conversation with my therapist. She gave me some really great concepts to think about with cognitive behavioral therapy. And it was Wednesday, and my next appointment isn’t until next Thursday. And I got in this huge fight with my wife, and the framework doesn’t make sense to me. I’m distorted and now I’ve got the best trained CBT AI tool right next to me, giving me great counsel on the situation and what I can do about it, and really saving the moment and saving a series of micro steps that improve mental health over time.
So is that startup perfect? No. Do we have to trust the model that it’s trained on? Yes. Do we have to have the checks and balances on the model that is trained on? Yes. Should we do it? Absolutely.
And we also need that supervision in there. When someone walks in and says, how do I commit suicide? Because I’m going to do it tonight. And if the model is hallucinating, it has to stop itself, which it won’t and shouldn’t because it’s trained right. But if it would, there has to be a measure intact where there will be no human life lost using GPT. I buy the things that it told it back. We can’t control human behavior at the end of the day, but the model itself shouldn’t be losing it and then training someone to do something we don’t want it to do. So I think the startups are absolutely mandatory and we have to proceed with caution. But if you left it to health systems or the health institutions by themselves, we’ll never see the pace we need to see to get the the systems to change at the level we need them to change.
SY: Thank you. And that is unfortunately we have to take we have to stop. That’s all we have time for today. And I know that we could sit here for so much longer. There’s so many more questions, but thank you to all of our panelists for taking the time out of your day to enlighten all of us. It’s been a really good conversation. Thank you. Thank you.
Thanks to our sponsors:
CALIFORNIA HEALTH CARE FOUNDATION, THE TRIBAL ALLIANCE OF SOVEREIGN INDIAN NATIONS, WESTERN STATES PETROLEUM ASSOCIATION, PHYSICIAN ASSOCIATION OF CALIFORNIA; KP PUBLIC AFFAIRS, PERRY COMMUNICATIONS, CAPITOL ADVOCACY, LUCAS PUBLIC AFFAIRS, THE WEIDEMAN GROUP, and CALIFORNIA PROFESSIONAL FIREFIGHTERS
Want to see more stories like this? Sign up for The Roundup, the free daily newsletter about California politics from the editors of Capitol Weekly. Stay up to date on the news you need to know.
Sign up below, then look for a confirmation email in your inbox.
Leave a Reply